Understand the power of machine learning within the context of gym management and how it can be used to transform your member data into actionable insights
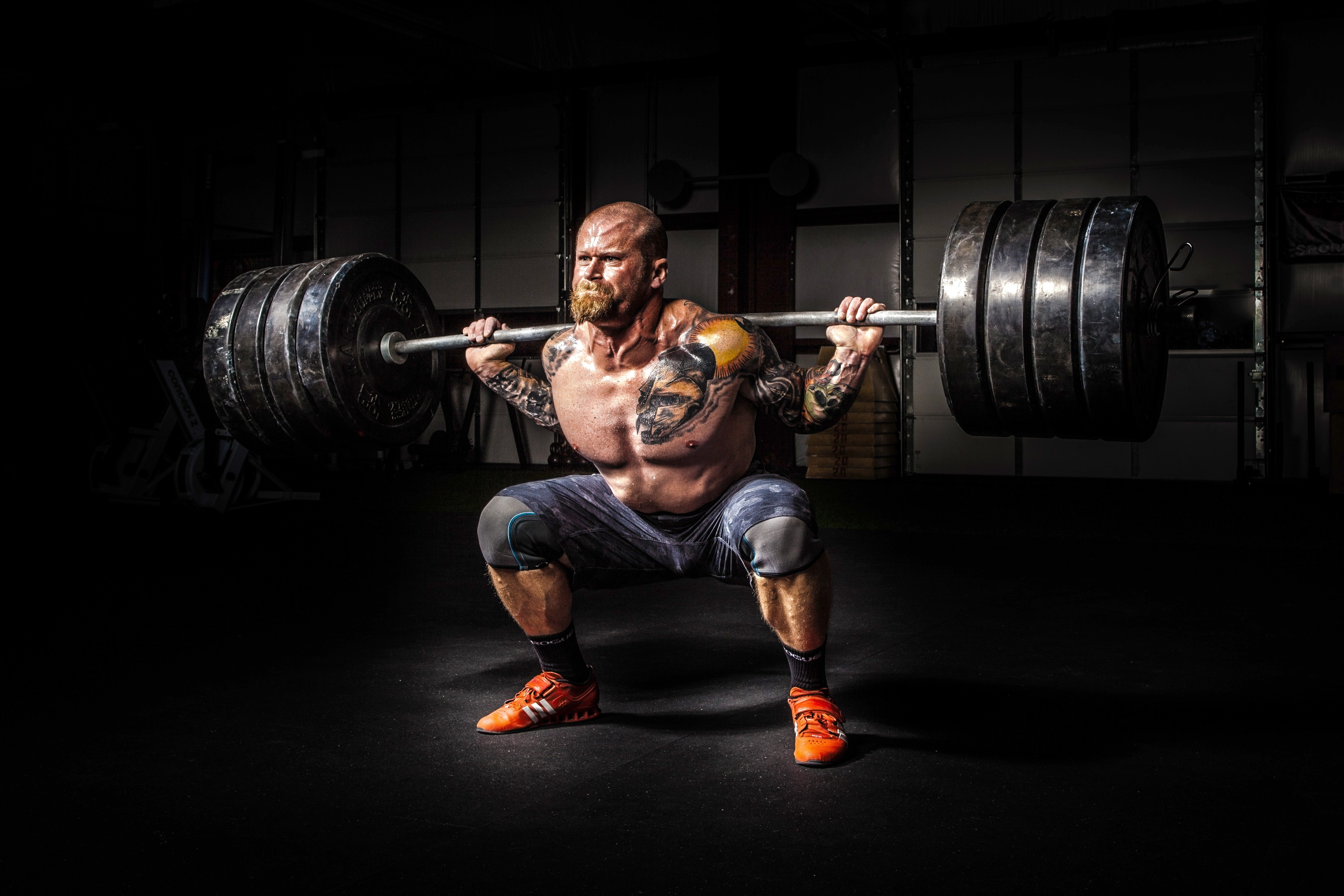
Understand the power of machine learning within the context of gym management and how it can be used to transform your member data into actionable insights
If I were to ask you what the biggest factors for members leaving your gym were, what would you tell me?
It’s usually a combination of different factors, most of which are never spoken about out loud or mentioned in exit surveys by members.
Most of the time though, it’s simply a loss in motivation.
While there is no metric for measuring a loss in motivation, there are a ton of factors that members display--even unconsciously--that can let you know that they’re losing motivation or are at-risk to churn.
Identifying these factors isn’t easily done by people or even the best data analysts because there is just so much data to process and look at that it’s almost impossible.
However, with machine learning you can choose certain data sets concerning your members and their behaviors for computers to process and ultimately assess a client’s churn-risk and engage them correctly for better gym member retention.
Artificial Intelligence (AI) is the process of using a complex set of algorithms to make decisions based on data that is mined, learned from, and processed.
Machine learning is a vital component of AI used to inform the decisions made through finding correlations between different and often times unrelated datasets.
Ultimately, Machine Learning acts as the analyst, and AI the engine that takes action based on the data.
How this differs from business to business depends on the datasets involved, the outcomes, and the actions taken. Here we’ll look at an example of how Target used machine learning in one of their online marketing campaigns.
When Andrew Pole of Target was asked by his marketing colleagues if he could correctly predict a woman’s pregnancy based on purchasing behaviors, he gladly took up the challenge.
Target, like many businesses in the fitness industry, understood that retaining clients is vastly less expensive than acquiring new ones.
They wanted to target new expecting mothers to purchase all of their maternity products as they were very likely to continue to make purchases there as a one-stop solution for all of their shopping needs.
So Pole used machine learning to assign a likelihood that a woman was pregnant based on her purchase decisions. They found expecting mothers often purchased things like large purses to double as diaper bags, specific lotions, supplements, and other things typical of expecting mothers to purchase.
Using this data they would assign a likelihood to each client and if they met or surpassed a percentage of this threshold then they would enter a campaign in which they were supplied with coupons for maternity products.
The algorithm was so accurate that an angry father approached one of the managers within a Target retail store and demanded to know why his daughter had been receiving coupons for maternity products when she wasn’t even pregnant.
The manager profusely apologized and followed-up to apologize again a few days later only to be told by the father that he was mistaken and his daughter actually was pregnant.
As you can imagine, Target saw huge results from the campaign and many other companies are using predictive analytics powered by machine learning to direct their marketing and sales campaigns.
As noted earlier, every gym has a trove of customer data that they utilize for different reasons. When it comes to using different data sets for retention purposes, the process is usually looked at from an attendance perspective.
While attendance is definitely a strong signal, it can be misleading if it’s the only signal you’re paying attention to.
That’s why it’s important that you take into account as many different data points as possible to better understand why people left your gym.
Some data points that can be taken into consideration for churn-risk assessment include:
These are just a few of the data points that can be mined for determining specific outcomes related to churn. In most cases, there are hundreds of factors that go into determining an at-risk percentage; however, for the sake of this article we’ll use examples that take from just a few.
When using predictive models, it’s important to define as many characteristics as possible that will, when counted all together, predict a likelihood of that outcome.
For example, we all know what an apple looks like. However, if we wanted to train a computer to predict the likelihood that something is an apple, we would have to outline its characteristics first:
If there’s an object that contains ¾ of these attributes, then we would determine that it’s 75% likely to be an apple.
Now defining a certain outcome for churn is a bit different as there is no one-size-fits-all list of attributes that determine if someone will churn.
However, machine learning can process an array of factors and behaviors that were prevalent in past churned members, find correlations, and use those correlations to assign probabilities to existing members.
For example, let’s say that a large amount of members who churned in the past had the following data points:
The algorithm would mark these behaviors as being highly prevalent among members with these data points and assign a higher churn probability to future members that display these attributes and behaviors.
This is why it’s important to maximize your data set input or beacons. In other words, the more data you have concerning your members, the more correlations the Business Intelligence module will be able to find.
Now that doesn’t mean you need to ask them unnecessary or irrelevant questions.
Simply placing some get-to-know them questions in the signup process will suffice and machine learning can withdraw behavioral data points once they start interacting with your apps, machines, website, or even front desk.
Once you have your beacons set up to withdraw data, machine learning will then learn about your gym members and begin predicting their churn-risk percentages based on the data provided.
Then you can automate the correspondence you want to send to segmented members that are past a specified threshold of at-risk percentage to keep them engaged and prevent churn.
All the data and insights in the world won’t be worth much unless there’s an automated campaign behind it to foster results.
For example, users can be segmented by their churn-risk and be given specific offers through targeted messaging to ensure the highest chance of engagement and retention.
Netflix does exactly this with their predictive analytics and automation. Based on what I’ve watched and people like me have watched, they know what content to add and send me messages about the content with the subject line: “Adam, we just added a movie you might like”.
This isn’t manual. It’s an automated message they send to thousands of people based on their content predilections. For other people, it may be a different move, show, or even have a different subject line.
Gyms can do this as well. You create variety of messages for each situation and automate them to be sent to your members.
For clients that may be close to churning, this message can be an offer for a highly reduced or free personal training package, a reduced contract extension, or even free merchandise that needs to be picked up at the gym so they’re encouraged to workout.
Simply establish the rules that users need to meet for this criteria and the messages will dynamically populate users into the campaign based on their attributes and the events that have occurred.
AI will begin to assess which ones are the best performing ones and from there automate them depending on the situation, customer, and other data that will ensure the message a user gets is as targeted as possible.
Once you create content for each situation you feel may occur or warrant a message, the specified users that fit into these criteria will be sent the right message.
Thus, with all of the analytics behind automation, you can increase your retention rates based on correlations of data that would be otherwise impossible to find.
Discover how our innovative solutions can elevate your business. Request a demo today and see the difference firsthand. Let's grow, together!